User-defined functions: summarizing noise data
This example works with a model of sound levels for the United States produced by the US National Park Service. We want to compute the average noise level for each municipality in Hawaii, but the standard mean
operation provided by exactextract is not appropriate for the logarithmic decibel scale used for sound levels.
To avoid working with large data files, we use the sound level grid provided for Hawaii, along with the TIGER shapefile of 2023 municipal boundaries (“county subdivisions”) published by the US Census Bureau:
[1]:
sound_fname = 'hawaii/HI_L50dBA_sumDay_exi.tif'
municipalities_fname = 'hawaii/tl_2023_15_cousub.zip'
The sound boundaries are published in UTM 4N (meters), while the municipal boundaries use NAD 1983 geographic coordinates. Rather than pass the filenames to exact_extract
, we will first load the datasets and reproject the municipal boundaries to the UTM coordinate system used by the sound grid.
[2]:
from exactextract import exact_extract
from matplotlib import pyplot
import rasterio
import rasterio.plot
import geopandas as gpd
sound_ds = rasterio.open(sound_fname)
municipalities = gpd.read_file(municipalities_fname).to_crs(sound_ds.crs)
fig, ax = pyplot.subplots()
ax.set_xlim([0.3e6, 1.0e6])
ax.set_ylim([2.0e6, 2.5e6])
rasterio.plot.show(sound_ds, ax=ax)
municipalities.plot(ax=ax, facecolor='none', edgecolor='black', linewidth=0.2)
[2]:
<Axes: >
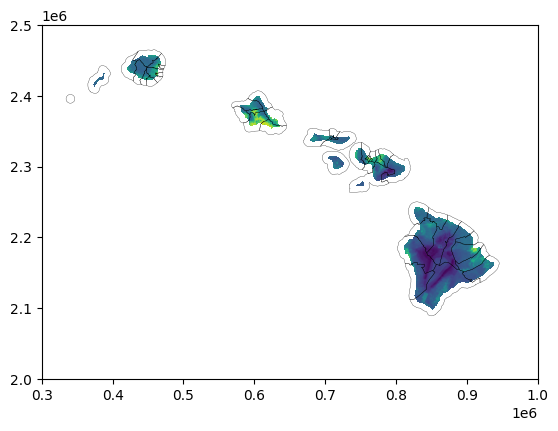
The following function can be used to compute the mean sound levels:
[3]:
import numpy as np
def mean_sound_db(values, coverage):
# transform values, take average, transform result
values = np.power(10, values)
mean = np.average(values, weights=coverage)
return np.log10(mean)
The user-defined function can now be used in the same way as a built-in summary operation. Unsurprisingly, we see that Honolulu is the loudest municipality in Hawaii.
[4]:
results = exact_extract(sound_ds, municipalities, mean_sound_db, include_cols=['GEOID', 'NAME'], output='pandas')
results.sort_values(by=['mean_sound_db'], ascending=False)
[4]:
GEOID | NAME | mean_sound_db | |
---|---|---|---|
11 | 1500390810 | Honolulu | 50.403354 |
33 | 1500390270 | Ewa | 49.420535 |
34 | 1500990900 | Kahului | 49.416380 |
32 | 1500393420 | Wahiawa | 49.414049 |
13 | 1500391800 | Koolaupoko | 48.554184 |
5 | 1500993060 | Puunene | 47.855280 |
37 | 1500993870 | Wailuku | 47.531631 |
21 | 1500190630 | Hilo | 47.491786 |
3 | 1500792160 | Lihue | 47.213052 |
42 | 1500993330 | Spreckelsville | 47.006436 |
12 | 1500393600 | Waianae | 46.891559 |
30 | 1500992250 | Makawao-Paia | 46.169428 |
7 | 1500791080 | Kapaa | 46.048761 |
28 | 1500792970 | Puhi-Hanamaulu | 45.986987 |
4 | 1500793780 | Wailua-Anahola | 45.556824 |
29 | 1500990360 | Haiku-Pauwela | 45.062474 |
19 | 1500192610 | North Kona | 44.449442 |
31 | 1500993690 | Waihee-Waikapu | 44.227507 |
41 | 1500991980 | Lahaina | 43.614811 |
10 | 1500393510 | Waialua | 43.020145 |
1 | 1500790180 | Eleele-Kalaheo | 42.995253 |
9 | 1500391710 | Koolauloa | 42.980143 |
2 | 1500791260 | Kaumakani-Hanapepe | 42.904206 |
39 | 1500991530 | Kihei | 42.618990 |
6 | 1500790540 | Hanalei | 42.488026 |
27 | 1500791620 | Koloa-Poipu | 42.177167 |
35 | 1500991890 | Kula | 42.043837 |
26 | 1500791440 | Kekaha-Waimea | 41.944628 |
14 | 1500190720 | Honokaa-Kukuihaele | 41.780611 |
40 | 1500990090 | East Molokai | 41.377057 |
22 | 1500191350 | Keaau-Mountain View | 41.020687 |
20 | 1500193150 | South Kohala | 40.443613 |
15 | 1500192520 | North Kohala | 40.338913 |
25 | 1500192880 | Papaikou-Wailea | 39.910005 |
16 | 1500192790 | Pahoa-Kalapana | 39.805841 |
36 | 1500992070 | Lanai | 39.660176 |
17 | 1500193240 | South Kona | 39.441267 |
24 | 1500192700 | Paauhau-Paauilo | 39.416518 |
43 | 1500993960 | West Molokai | 39.159257 |
38 | 1500990450 | Hana | 39.091584 |
0 | 1500590990 | Kalawao | 38.723797 |
23 | 1500192430 | North Hilo | 38.371793 |
18 | 1500191170 | Kau | 37.412745 |
8 | 1500792340 | Niihau | 35.633805 |